AI in Healthcare: Tools for Efficient and Accurate Disease Detection
The advent of Artificial Intelligence (AI) in healthcare has ushered in a new era of medical innovation, particularly in the realm of disease detection. By leveraging advanced algorithms and machine learning, AI has proven to be a powerful tool in identifying diseases with unprecedented accuracy and efficiency. This comprehensive article explores the various AI tools used in disease detection, highlights success stories, and delves into the future implications of this transformative technology.
Introduction to AI in Disease Detection
AI’s role in healthcare extends beyond administrative tasks to critical areas like disease detection. By analyzing vast datasets, AI systems can identify patterns and anomalies that might be missed by human practitioners. This capability is particularly beneficial in early disease detection, where timely intervention can significantly improve patient outcomes.
Key AI Tools in Disease Detection
Several AI tools have been developed to enhance disease detection capabilities. These tools utilize various machine learning techniques, including neural networks, natural language processing, and image recognition. Here are some of the most impactful AI tools in disease detection:
- Deep Learning Algorithms: These algorithms analyze medical images (e.g., X-rays, MRIs) to detect abnormalities with high precision.
- Natural Language Processing (NLP): NLP algorithms process and interpret unstructured medical data, such as patient records and clinical notes, to identify potential health issues.
- Predictive Analytics: By examining historical health data, predictive analytics tools can forecast disease outbreaks and individual patient risks.
Success Stories and Exemplary Cases
AI in disease detection is not just a theoretical concept; it has already demonstrated significant real-world success. Here are some notable examples:
- IBM Watson Health: Known for its advanced AI capabilities, IBM Watson has been instrumental in cancer detection, offering diagnostic recommendations based on a comprehensive analysis of medical literature and patient data.
- Google Health: Utilizing deep learning, Google Health’s AI systems have shown remarkable accuracy in detecting diabetic retinopathy, a condition that can lead to blindness if not diagnosed early.
- Arterys: This company leverages AI for advanced medical imaging analysis, significantly improving the detection of cardiovascular diseases.
AI in Radiology
Radiology has been one of the most impactful areas of AI application in healthcare. AI-powered radiology tools can analyze medical images more quickly and accurately than human radiologists, leading to faster diagnoses and treatment plans.
For instance, Zebra Medical Vision uses AI algorithms to detect a range of conditions from medical imaging data, such as liver disease, lung abnormalities, and bone density issues. This tool has been pivotal in providing early and accurate diagnoses, reducing the burden on radiologists and improving patient care.
AI in Pathology
Pathology, the study of disease through the examination of organs, tissues, and bodily fluids, has greatly benefited from AI integration. AI tools can scan and analyze pathological samples with a high degree of accuracy, identifying cancerous cells and other abnormalities.
Companies like PathAI are leading the way in this field. PathAI’s algorithms assist pathologists in diagnosing diseases more accurately and efficiently, providing critical support in the fight against cancer and other serious conditions.
AI in Genomics
Genomics involves the analysis of an individual’s genetic material to understand diseases at a molecular level. AI has revolutionized this field by enabling the rapid processing of genetic data to identify mutations and predict disease risks.
An example is Freenome, a company that uses AI to detect early-stage cancer by analyzing blood samples. Their AI platform combines machine learning with genomics to uncover patterns associated with cancer, leading to early and potentially life-saving interventions.
Challenges and Ethical Considerations
While the benefits of AI in disease detection are substantial, there are challenges and ethical considerations that need to be addressed. These include:
- Data Privacy: Ensuring patient data is securely stored and used ethically.
- Bias in AI Algorithms: Addressing biases that may exist in AI training data, which can lead to inaccurate diagnoses for certain demographic groups.
- Regulatory Compliance: Navigating the complex regulatory landscape to ensure AI tools meet healthcare standards and regulations.
To mitigate these challenges, ongoing collaboration between AI developers, healthcare providers, and regulatory bodies is essential. This collaboration will help ensure that AI tools are safe, effective, and equitable for all patients.
The Future of AI in Disease Detection
The future of AI in disease detection looks promising, with continuous advancements in technology and growing adoption in healthcare settings. Future developments may include:
- Enhanced Diagnostic Accuracy: Continued improvement in AI algorithms to increase diagnostic accuracy across a broader range of diseases.
- Personalized Medicine: Utilizing AI to tailor treatments based on individual patient profiles, leading to more effective and personalized healthcare.
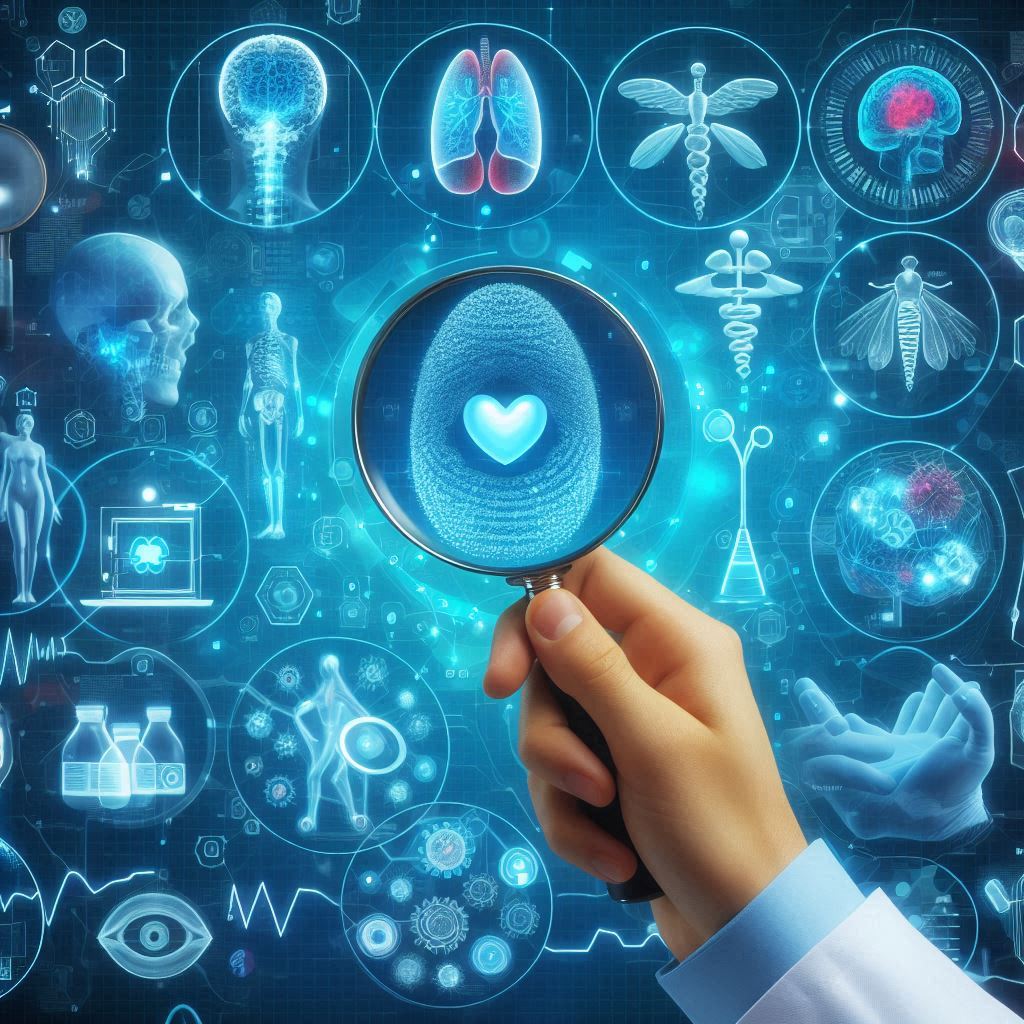
Pros and Cons of AI in Healthcare: Tools for Efficient and Accurate Disease Detection
Artificial Intelligence (AI) has revolutionized various sectors, and healthcare is no exception. The integration of AI in disease detection offers significant advantages, but it also comes with certain drawbacks. This article outlines the key pros and cons of using AI tools in healthcare for disease detection, providing a balanced view of this transformative technology.
Pros of AI in Disease Detection
The benefits of AI in healthcare are numerous and multifaceted, enhancing the accuracy, efficiency, and accessibility of medical services.
1. Enhanced Diagnostic Accuracy
AI algorithms can analyze medical images and data with a high degree of precision, often surpassing human capabilities. This leads to more accurate diagnoses, particularly in detecting complex diseases like cancer and neurological disorders.
2. Early Disease Detection
AI tools can identify early signs of diseases that might be overlooked by human eyes. Early detection is crucial for diseases such as cancer, where timely intervention can significantly improve survival rates.
3. Efficiency and Speed
AI systems can process and analyze large volumes of medical data quickly, reducing the time needed for diagnosis. This efficiency helps in managing patient loads and speeding up the treatment process.
4. Predictive Analytics
By analyzing historical and real-time data, AI can predict disease outbreaks and patient risk profiles. This predictive capability allows for proactive healthcare measures, potentially preventing disease progression.
5. Cost-Effective
AI can help reduce healthcare costs by minimizing diagnostic errors, reducing unnecessary tests, and streamlining operations. Efficient disease detection and management can lead to significant savings for healthcare providers and patients.
Cons of AI in Disease Detection
Despite its many advantages, the use of AI in healthcare also presents several challenges and drawbacks that need to be carefully considered.
1. Data Privacy Concerns
The use of AI requires access to vast amounts of patient data, raising concerns about data security and privacy. Ensuring that patient information is protected and used ethically is a significant challenge.
2. Bias in AI Algorithms
AI systems are only as good as the data they are trained on. If the training data contains biases, the AI can perpetuate and even exacerbate these biases, leading to inaccurate diagnoses for certain demographic groups.
3. Lack of Transparency
AI algorithms can be complex and opaque, making it difficult for healthcare professionals to understand and trust the results. This lack of transparency can hinder the adoption of AI tools in clinical settings.
FAQs on AI in Healthcare: Tools for Efficient and Accurate Disease Detection
Artificial Intelligence (AI) in healthcare is transforming the way diseases are detected and diagnosed. This FAQ section addresses common questions about the role of AI in disease detection, its benefits, challenges, and future prospects.
1. What is AI in healthcare?
AI in healthcare refers to the use of artificial intelligence technologies, such as machine learning, natural language processing, and deep learning, to analyze medical data and assist in diagnosing, treating, and predicting diseases. AI tools can process vast amounts of data quickly and accurately, providing valuable insights that improve patient care.
For more information, visit IBM Watson Health.
2. How does AI improve disease detection?
AI improves disease detection by analyzing medical images, genetic data, and patient records to identify patterns and anomalies. It can detect diseases at earlier stages, provide more accurate diagnoses, and reduce the likelihood of human error. AI tools like deep learning algorithms and natural language processing enhance the ability to interpret complex medical data.
Learn more about AI’s impact on disease detection at Google Health Research.
3. What are some examples of AI tools used in disease detection?
Examples of AI tools used in disease detection include:
- Deep Learning Algorithms: Analyze medical images like X-rays and MRIs.
- Natural Language Processing (NLP): Interpret unstructured data from medical records.
- Predictive Analytics: Forecast disease outbreaks and individual patient risks.
For a detailed list of AI tools, visit Arterys.
4. What are the benefits of using AI in disease detection?
The benefits of using AI in disease detection include:
- Enhanced Diagnostic Accuracy: AI can detect diseases more accurately than traditional methods.
- Early Disease Detection: AI identifies diseases at earlier stages, leading to better treatment outcomes.
- Efficiency and Speed: AI processes large datasets quickly, speeding up diagnosis and treatment.
- Predictive Capabilities: AI predicts disease risks and outbreaks, enabling proactive healthcare measures.
- Cost Savings: AI reduces diagnostic errors and unnecessary tests, lowering healthcare costs.
Read more about the benefits at PathAI.
5. What are the challenges and limitations of AI in disease detection?
Despite its advantages, AI in disease detection faces several challenges:
- Data Privacy: Protecting patient data and ensuring its ethical use.
- Bias in Algorithms: Addressing biases in training data that can affect accuracy for certain groups.
- Transparency: Making AI algorithms understandable and trustworthy for healthcare professionals.
- Regulatory Compliance: Meeting regulatory standards for safety and effectiveness.
- Dependence on Technology: Balancing AI assistance with human oversight to avoid over-reliance.
Explore these challenges further at Freenome.
6. How is AI addressing the issue of bias in healthcare?
AI addresses bias in healthcare by using diverse and representative datasets for training algorithms. Regular audits and updates of AI systems help ensure fair and accurate performance across different demographic groups. Collaboration between AI developers and healthcare providers is essential to mitigate biases and enhance algorithmic fairness.
Find out more about efforts to reduce bias in AI at Zebra Medical Vision.
7. What role do regulatory bodies play in AI healthcare applications?
Regulatory bodies play a crucial role in ensuring the safety, effectiveness, and ethical use of AI in healthcare. They develop guidelines and standards that AI tools must meet to be approved for clinical use. Ongoing collaboration with regulatory agencies helps address emerging challenges and ensures that AI technologies benefit patients while maintaining high standards of care.
Learn about regulatory frameworks for AI in healthcare at FDA AI/ML Medical Devices.
8. How can healthcare providers integrate AI into their practice?
Healthcare providers can integrate AI into their practice by:
- Training Staff: Providing training on AI tools and their applications in clinical settings.
- Collaboration: Working with AI developers to tailor solutions to specific healthcare needs.
- Investing in Technology: Acquiring and implementing AI-powered systems for diagnostics and patient management.
- Maintaining Human Oversight: Ensuring AI complements rather than replaces human expertise, with healthcare professionals overseeing AI-assisted decisions.
For more insights on integrating AI, visit Healthcare IT News.
9. What is the future of AI in disease detection?
The future of AI in disease detection is promising, with potential advancements including:
- Improved Diagnostic Accuracy: Continuous refinement of AI algorithms to enhance accuracy across diverse diseases.
- Personalized Medicine: AI-driven personalized treatment plans based on individual genetic profiles and health data.
- Real-time Monitoring: Integration with wearable devices for continuous health monitoring and early detection of issues.
- Global Health Applications: Expanding AI tools to under-resourced regions, improving global health outcomes.
Discover more about the future trends in AI and healthcare at McKinsey & Company.
Disclaimer and Caution Regarding AI in Healthcare
The following disclaimer and cautionary statements are provided to ensure a clear understanding of the limitations, risks, and responsibilities associated with the use of Artificial Intelligence (AI) in healthcare, particularly in the context of disease detection and diagnosis. It is crucial for healthcare providers, patients, and stakeholders to be aware of these considerations to make informed decisions.
Disclaimer
The information provided herein is for educational and informational purposes only. It is not intended to serve as medical advice, diagnosis, or treatment. AI tools are designed to assist healthcare professionals by providing insights and data analysis; however, they are not a substitute for professional medical judgment, expertise, and individualized patient care.
1. Accuracy and Reliability
While AI technologies have demonstrated significant potential in improving diagnostic accuracy, it is important to recognize that no AI system is infallible. The accuracy of AI-driven diagnostics can vary based on the quality and diversity of the training data, the specific algorithms used, and the clinical context. Healthcare professionals should critically evaluate AI-generated insights and integrate them with their clinical knowledge and experience.
2. Continuous Improvement
AI technologies are continuously evolving, with ongoing research and development aimed at enhancing their performance. As a result, AI tools currently in use may be subject to updates and improvements. Users should ensure that they are utilizing the most up-to-date versions of AI tools and remain informed about advancements in the field.
3. Ethical Use
The ethical use of AI in healthcare is paramount. AI tools should be used in accordance with established ethical guidelines and principles, ensuring that patient data is handled with the utmost care and confidentiality. It is essential to obtain informed consent from patients when their data is used for AI-driven analysis and to be transparent about the use of AI in their care.
Cautionary Statements
The integration of AI in healthcare, particularly in disease detection and diagnosis, involves several cautionary considerations that must be acknowledged and addressed to mitigate risks and ensure patient safety and trust.
1. Data Privacy and Security
The use of AI in healthcare necessitates the collection and analysis of vast amounts of patient data. This raises significant concerns about data privacy and security. Healthcare providers must implement robust data protection measures to safeguard patient information from unauthorized access, breaches, and misuse. Compliance with data protection regulations, such as HIPAA (Health Insurance Portability and Accountability Act) and GDPR (General Data Protection Regulation), is essential.
2. Algorithmic Bias and Fairness
AI systems are only as good as the data they are trained on. If the training data contains biases, these biases can be perpetuated and even amplified by AI algorithms, leading to disparities in diagnostic accuracy across different demographic groups. Developers and users of AI tools must actively work to identify and address biases, ensuring that AI-driven diagnostics are fair and equitable for all patients.
3. Transparency and Explainability
AI algorithms can be complex and difficult to interpret, making it challenging for healthcare professionals to understand how decisions are made. Lack of transparency and explainability can undermine trust in AI tools. It is crucial to develop and use AI systems that provide clear, understandable explanations for their recommendations, enabling healthcare professionals to make informed decisions and communicate effectively with patients.
4. Regulatory Compliance
The regulatory landscape for AI in healthcare is still evolving. Ensuring that AI tools meet regulatory standards for safety, efficacy, and ethical use is a complex process. Healthcare providers must stay informed about regulatory requirements and ensure that the AI technologies they use comply with these standards. Collaboration with regulatory bodies can help address emerging challenges and ensure the responsible deployment of AI in healthcare.
5. Human Oversight
While AI can significantly enhance diagnostic capabilities, it is essential to maintain human oversight in the diagnostic process. AI tools should be used to support, not replace, the expertise and judgment of healthcare professionals. Ensuring that critical diagnostic decisions involve human review and validation helps mitigate the risks of AI errors and reinforces the importance of clinical expertise.
6. Training and Education
The effective use of AI in healthcare requires adequate training and education for healthcare professionals. Understanding how AI tools work, their limitations, and how to interpret their outputs is essential for integrating AI into clinical practice. Continuous education and training programs can help healthcare providers stay up-to-date with advancements in AI technology and best practices for its use.
7. Integration Challenges
Integrating AI tools into existing healthcare systems and workflows can be challenging. Compatibility issues, data integration, and workflow adjustments must be carefully managed to ensure that AI tools enhance rather than disrupt clinical practice. Healthcare organizations should develop comprehensive implementation plans and collaborate with AI developers to address integration challenges effectively.
Conclusion
AI in healthcare holds tremendous potential for improving disease detection and patient outcomes. However, it is essential to approach the use of AI with caution, recognizing its limitations and addressing the associated risks. By adhering to ethical guidelines, ensuring data privacy, mitigating biases, and maintaining human oversight, healthcare providers can harness the power of AI responsibly and effectively. Continuous education, collaboration with regulatory bodies, and ongoing efforts to improve AI technologies will be key to realizing the full benefits of AI in healthcare while ensuring patient safety and trust.
6 thoughts on “AI in Healthcare”