AI in Medicine: Revolutionizing Diagnosis and Treatment
Artificial Intelligence (AI) is rapidly transforming the landscape of medicine, bringing forth innovative solutions that enhance diagnostic accuracy and improve treatment outcomes. This article delves into the various ways AI is revolutionizing the field of medicine, from early diagnosis to personalized treatment plans.
AI in Diagnostic Imaging
One of the most prominent applications of AI in medicine is in diagnostic imaging. AI algorithms can analyze medical images such as X-rays, MRIs, and CT scans with remarkable precision. Companies like GE Healthcare and IBM Watson Health are leading the charge in developing AI tools that assist radiologists in detecting anomalies such as tumors, fractures, and other critical conditions.
Table 1: AI Applications in Diagnostic Imaging
Company | Technology | Application |
---|---|---|
GE Healthcare | Deep Learning | Tumor Detection |
IBM Watson Health | Machine Learning | Image Analysis |
Philips Healthcare | AI Algorithms | Cardiac Imaging |
AI in Personalized Medicine
Personalized medicine is another area where AI is making significant strides. By analyzing large datasets of patient information, AI can help develop personalized treatment plans tailored to the unique genetic makeup and medical history of each individual. This approach is particularly beneficial in the treatment of complex diseases such as cancer.
Success Stories and Exemplary Cases
Numerous funded companies have emerged as trailblazers in their respective domains, rewriting the norms of medical innovation. From tech titans like Tempus and Freenome to disruptive innovators like 23andMe and Guardant Health, these success stories underscore the transformative power of AI in personalized medicine.
Table 2: Leading Companies in Personalized Medicine
Company | Technology | Application |
---|---|---|
Tempus | Genomic Sequencing | Cancer Treatment |
Freenome | AI Diagnostics | Early Cancer Detection |
23andMe | Genetic Testing | Personal Health Insights |
Guardant Health | Liquid Biopsy | Cancer Detection |
AI in Drug Discovery
AI is also revolutionizing drug discovery by significantly reducing the time and cost involved in bringing new drugs to market. AI models can analyze vast amounts of data to identify potential drug candidates and predict their effectiveness. Companies like Exscientia and Berg Health are at the forefront of this innovative approach.
Table 3: AI in Drug Discovery
Company | Technology | Application |
---|---|---|
Exscientia | AI Drug Design | Target Identification |
Berg Health | Machine Learning | Biomarker Discovery |
Insilico Medicine | Deep Learning | Drug Repurposing |
AI in Predictive Analytics for Patient Care
Predictive analytics powered by AI can foresee potential health issues and suggest preventive measures, improving patient care. Hospitals and healthcare providers are using AI to predict patient outcomes, readmission rates, and even potential outbreaks of diseases. Companies like HCA Healthcare and Cerner Corporation are implementing AI-driven predictive analytics to enhance patient care.
Table 4: AI in Predictive Analytics
Company | Technology | Application |
---|---|---|
HCA Healthcare | Predictive Algorithms | Patient Outcomes |
Cerner Corporation | Data Analytics | Readmission Rates |
Epic Systems | Machine Learning | Disease Outbreaks |
Ethical Considerations and Challenges
Despite the significant advancements, the integration of AI in medicine also
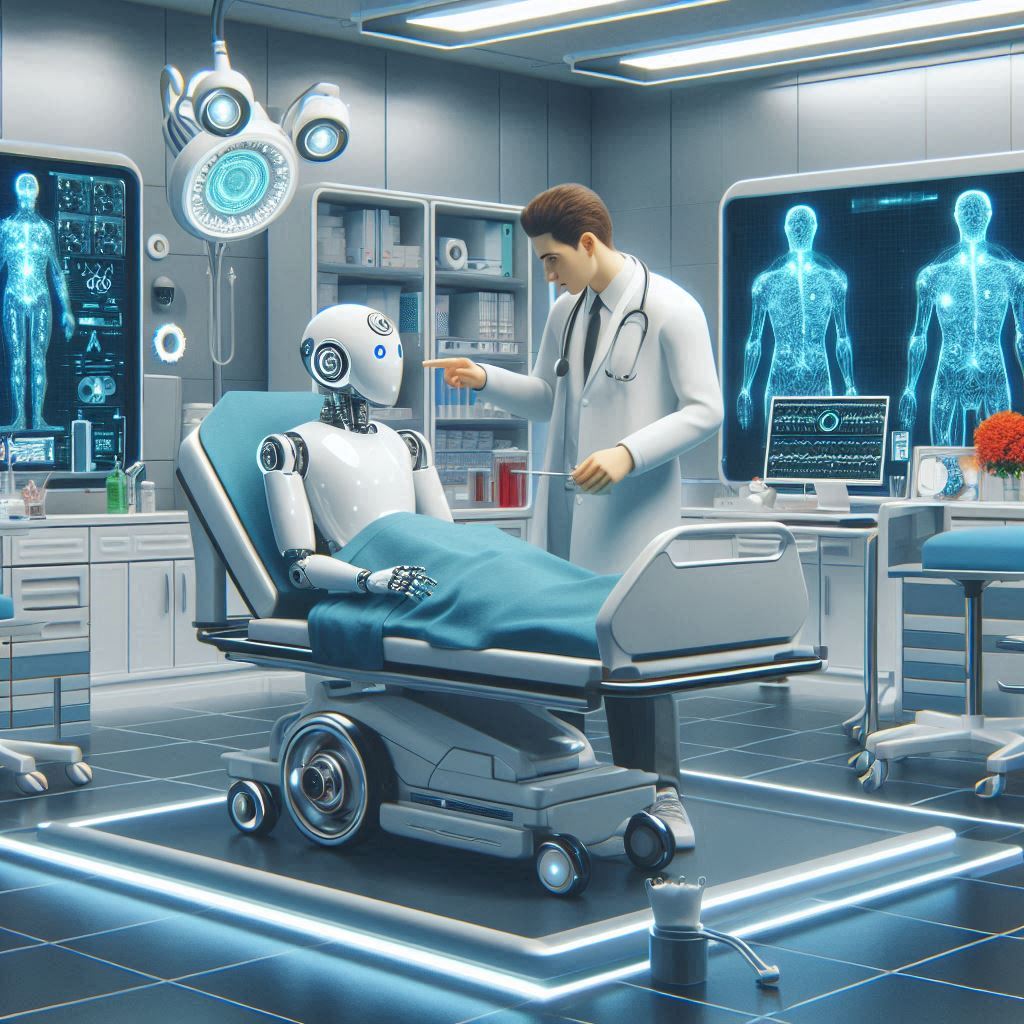
AI in Medicine: Revolutionizing Diagnosis and Treatment
Pros and Cons
Pros
- Improved Diagnostic Accuracy
- Enhanced Image Analysis: AI algorithms, such as deep learning, excel in analyzing medical images (e.g., X-rays, MRIs, CT scans) with high precision, often outperforming human radiologists.
- Early Detection: AI can identify subtle patterns and anomalies in data, facilitating the early detection of diseases like cancer, which is crucial for successful treatment.
- Personalized Treatment Plans
- Tailored Therapies: AI can analyze a patient’s genetic information, lifestyle, and medical history to develop personalized treatment plans, improving the effectiveness of therapies.
- Predictive Analytics: By analyzing vast amounts of patient data, AI can predict how patients will respond to different treatments, enabling more precise and individualized care.
- Efficiency and Cost Reduction
- Automation of Routine Tasks: AI can automate administrative tasks such as scheduling, billing, and documentation, freeing up healthcare professionals to focus on patient care.
- Resource Optimization: AI systems can optimize the allocation of medical resources, reducing waste and lowering healthcare costs.
- Enhanced Patient Monitoring
- Remote Monitoring: AI-powered devices and wearables can continuously monitor patients’ vital signs and alert healthcare providers to any significant changes, improving patient outcomes.
- Chronic Disease Management: AI can help manage chronic diseases by providing patients with personalized recommendations and monitoring their adherence to treatment plans.
- Accelerated Drug Discovery
- Predictive Modeling: AI can analyze biological data to identify potential drug candidates faster and more accurately than traditional methods.
- Clinical Trials Optimization: AI can optimize the design and execution of clinical trials, leading to more efficient and cost-effective drug development.
Cons
- Data Privacy and Security Concerns
- Sensitive Information: The use of AI in medicine involves handling large volumes of sensitive patient data, raising concerns about data breaches and privacy violations.
- Regulatory Compliance: Ensuring compliance with regulations such as HIPAA (Health Insurance Portability and Accountability Act) can be challenging when implementing AI systems.
- Bias and Fairness Issues
- Algorithmic Bias: AI algorithms can perpetuate and even exacerbate existing biases if they are trained on biased data, leading to unfair treatment of certain patient groups.
- Equity in Access: Not all healthcare facilities have equal access to advanced AI technologies, potentially widening the gap between different socio-economic groups.
- Reliability and Accountability
- Overreliance on AI: There’s a risk that healthcare professionals might become over-reliant on AI systems, potentially overlooking important clinical insights that only human judgment can provide.
- Error Accountability: Determining accountability for errors made by AI systems can be complex, raising legal and ethical questions.
- Technical and Implementation Challenges
- Integration with Existing Systems: Integrating AI with existing healthcare IT systems can be complex and costly.
- Continuous Learning Requirements: AI systems require continuous updates and learning to remain effective, necessitating ongoing maintenance and adaptation.
- Ethical and Moral Considerations
- Patient Consent: The use of AI in treatment decisions raises ethical questions about patient consent and the transparency of AI-driven decisions.
- Human Touch: AI-driven interactions may lack the empathy and personal touch that are essential in healthcare, potentially affecting patient satisfaction and trust.
Conclusion
AI has the potential to revolutionize medicine by improving diagnostic accuracy, personalizing treatments, enhancing efficiency, and accelerating drug discovery. However, it also brings challenges related to data privacy, bias, reliability, technical implementation, and ethical considerations. Balancing these pros and cons is essential for the successful and equitable integration of AI in healthcare.
1 thought on “AI in Medicine”