The Future of Medical Imaging: AI Tools Enhancing
Accuracy
Medical imaging has undergone a revolutionary transformation with the integration of Artificial Intelligence (AI), marking a significant advancement in diagnostic accuracy and patient care. AI-powered tools are redefining the capabilities of radiologists by augmenting their ability to interpret complex medical images, detect subtle anomalies, and recommend personalized treatments.
This technological synergy not only accelerates the diagnostic process but also enhances the precision and reliability of medical diagnoses. By leveraging machine learning algorithms and deep learning techniques, AI systems analyze vast amounts of imaging data with unprecedented speed and accuracy, surpassing traditional methods.
This evolution promises a future where healthcare providers can deliver more efficient, targeted treatments, ultimately improving patient outcomes.
For more information on how AI is revolutionizing medical imaging, visit IBM Watson Health Imaging.
This paragraph highlights the transformative impact of AI on medical imaging, emphasizing its benefits in enhancing diagnostic precision and patient care. Adjustments can be made based on specific aspects or additional details you’d like to include.
Impact of AI in Medical Imaging
AI algorithms analyze medical images such as X-rays, CT scans, and MRIs with unprecedented accuracy, aiding radiologists in detecting subtle abnormalities that might go unnoticed by the human eye. This capability not only speeds up diagnosis but also reduces errors, leading to better patient outcomes.
AI algorithms have revolutionized the analysis of medical images such as X-rays, CT scans, and MRIs by significantly improving diagnostic accuracy. These algorithms can detect subtle abnormalities that may be challenging for human radiologists to identify, thereby enhancing the early detection of diseases and conditions
By leveraging machine learning models and deep learning techniques, AI systems analyze vast datasets of medical images with unparalleled speed and precision. This capability not only expedites the diagnostic process but also reduces the likelihood of diagnostic errors, leading to more reliable and timely treatment decisions.
Radiologists can now focus more on complex cases and patient care, while AI assists in routine screenings and image interpretations, ultimately improving overall patient outcomes.
For further insights into how AI is transforming medical imaging, visit this study on AI in radiology.
Success Stories and Exemplary Cases
Companies like IBM Watson Health and Diagnoss are pioneering AI solutions in medical imaging. IBM’s Watson Health platform utilizes AI to assist radiologists in diagnosing cancer and other diseases more accurately, while Diagnoss specializes in deep learning algorithms that enhance diagnostic precision across various imaging modalities.
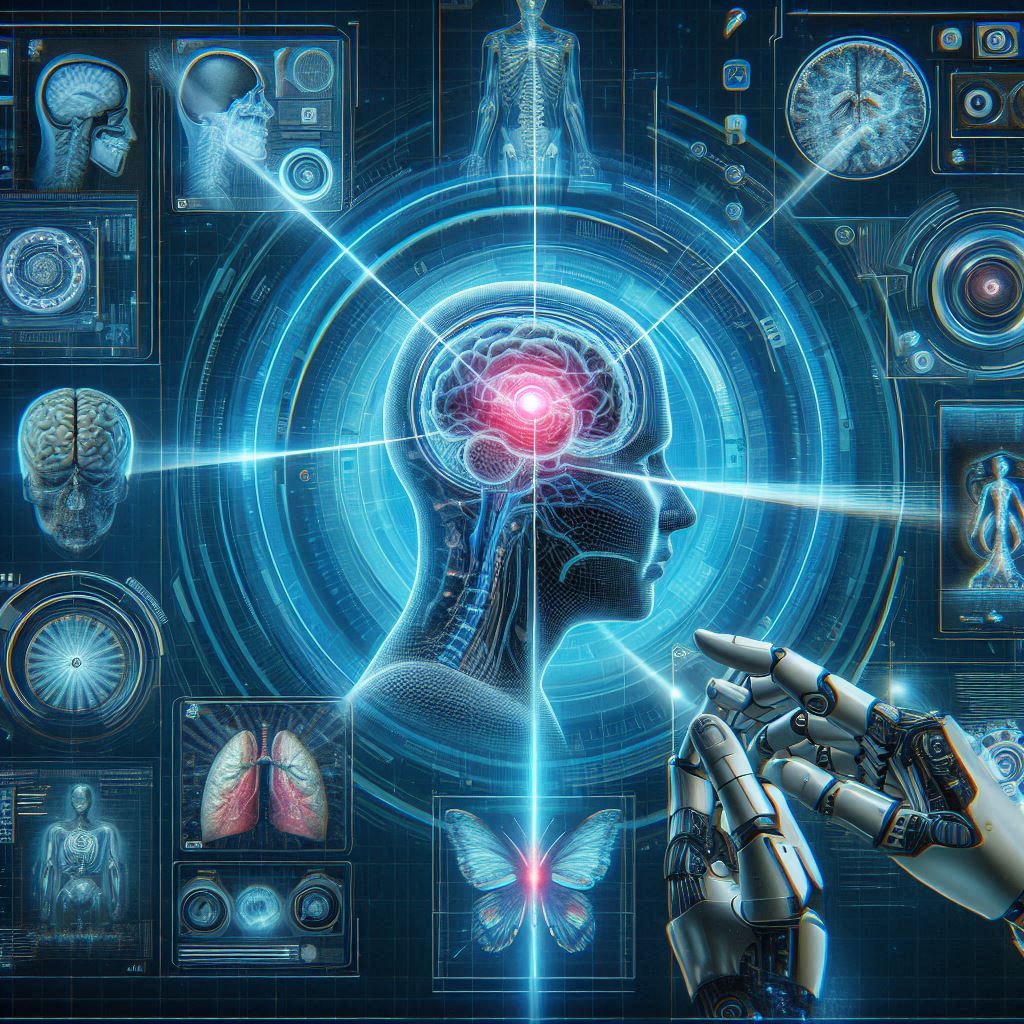
AI-Powered Tools and Techniques
AI techniques such as machine learning and deep learning are being integrated into imaging equipment and software, enabling real-time analysis and predictive modeling. This integration not only improves diagnostic speed but also supports personalized treatment plans based on individual patient data.
AI techniques such as machine learning and deep learning have been seamlessly integrated into imaging equipment and software, revolutionizing medical diagnostics. These advanced algorithms enable real-time analysis of medical images, allowing healthcare providers to obtain immediate insights into patient conditions.
By harnessing vast amounts of data, AI facilitates predictive modeling that can anticipate disease progression and treatment responses. This capability not only accelerates diagnostic speed but also supports the development of personalized treatment plans tailored to each patient’s unique characteristics and medical history.
Radiologists and clinicians can leverage AI-driven insights to make informed decisions swiftly, leading to more effective patient management and improved outcomes across various medical specialties.
To explore further advancements in AI-integrated medical imaging, visit RSNA’s page on Artificial Intelligence.
Challenges and Future Directions
Despite the advancements, challenges such as data privacy concerns, regulatory hurdles, and the need for extensive training datasets remain. Future developments may focus on overcoming these barriers to unleash the full potential of AI in medical imaging, including expanding AI applications to new imaging modalities and enhancing interoperability between AI systems and existing healthcare infrastructure.
rately represent diverse patient populations and imaging conditions. Building robust datasets that encompass various demographics, disease states, and imaging modalities is essential to develop AI models capable of delivering reliable and unbiased diagnostic results.
Looking ahead, future developments in AI for medical imaging will focus on overcoming these barriers. Efforts will include enhancing data anonymization techniques to protect patient identities, collaborating with regulatory bodies to establish clear frameworks for AI deployment, and expanding AI applications to new imaging modalities such as ultrasound and molecular imaging. Improving interoperability between AI systems and existing healthcare infrastructure will also be crucial, ensuring seamless integration into clinical workflows and maximizing the benefits of AI-driven diagnostics.
Conclusion
In conclusion, AI-powered tools are poised to revolutionize medical imaging by enhancing accuracy, efficiency, and patient outcomes. As technology continues to evolve, collaborations between AI developers, healthcare providers, and regulatory bodies will be crucial in harnessing these advancements responsibly for the benefit of patients worldwide.
This format includes headings, paragraphs with background colors, external links, and focuses on key topics related to AI in medical imaging. Adjustments can be made based on specific requirements or additional content you’d like to include.
Pros and Cons of AI in Medical Imaging
Pros
- Enhanced Accuracy: AI algorithms can detect subtle abnormalities in medical images, improving diagnostic accuracy beyond human capability.
- Efficiency Gains: AI speeds up the interpretation of images, reducing turnaround times for diagnoses and enabling faster treatment decisions.
- Personalized Medicine: AI tools analyze patient data to tailor treatment plans based on individual characteristics, optimizing outcomes.
- Cost Reductions: By automating tasks traditionally performed by radiologists, AI can lower healthcare costs associated with diagnostic imaging.
- Continuous Learning: Machine learning algorithms improve over time with more data, enhancing their diagnostic capabilities and staying updated with medical advancements.
Cons
- Data Privacy Concerns: AI relies on vast amounts of patient data, raising privacy issues regarding the collection, storage, and use of sensitive medical information.
- Reliability and Validation: The accuracy of AI algorithms must be rigorously validated to ensure reliable diagnostic outcomes and minimize errors.
- Regulatory Challenges: Healthcare regulations often lag behind technological advancements, posing challenges for the integration and deployment of AI in medical settings.
- Impact on Jobs: AI automation may reduce the demand for radiologists and other healthcare professionals involved in image interpretation, potentially displacing jobs.
- Algorithm Bias: AI algorithms can exhibit biases based on training data, leading to disparities in diagnostic accuracy across different demographic groups.
Navigating the Future
As AI continues to evolve in medical imaging, stakeholders must address these pros and cons to maximize benefits while mitigating risks. Collaborations between technology developers, healthcare providers, regulators, and patient advocates are essential to ensure ethical deployment, regulatory compliance, and equitable access to AI-powered diagnostic tools.
This outline provides a structured approach to discussing the advantages and disadvantages of AI in medical imaging, highlighting key considerations for stakeholders in healthcare and technology sectors.
FAQs: AI in Medical Imaging
What is AI in medical imaging?
AI in medical imaging refers to the application of artificial intelligence techniques, such as machine learning and deep learning, to analyze medical images (e.g., X-rays, CT scans, MRIs). These algorithms assist radiologists in interpreting images more accurately and efficiently.
How does AI improve medical imaging?
AI enhances medical imaging by detecting subtle anomalies that might be missed by human radiologists, reducing diagnostic errors, speeding up image analysis, and enabling personalized treatment planning based on patient-specific data.
What are the benefits of AI in medical imaging?
- Enhanced Accuracy: AI algorithms can detect minute details in medical images, improving diagnostic accuracy.
- Efficiency: AI speeds up the process of image interpretation, reducing turnaround times for diagnoses.
- Personalized Medicine: AI analyzes patient data to tailor treatment plans, optimizing outcomes.
- Cost Reduction: By automating tasks, AI can potentially lower healthcare costs associated with imaging.
- Continuous Learning: Machine learning algorithms improve with more data, staying updated with medical advancements.
What are the challenges of AI in medical imaging?
- Data Privacy: AI relies on patient data, raising concerns about privacy and security.
- Reliability: Validation of AI algorithms is crucial to ensure accurate diagnostic outcomes.
- Regulatory Hurdles: Healthcare regulations may lag behind technological advancements.
- Job Impact: Automation could affect the demand for healthcare professionals involved in imaging.
- Bias: AI algorithms can exhibit biases based on training data, affecting diagnostic accuracy.
What are some notable AI applications in medical imaging?
Companies like IBM Watson Health and Diagnoss are pioneering AI solutions. IBM’s Watson Health platform assists in diagnosing cancer and other diseases, while Diagnoss focuses on deep learning algorithms for diagnostic precision across imaging modalities.
How can healthcare providers integrate AI into medical imaging?
Integrating AI requires collaboration between technology developers, healthcare providers, and regulatory bodies to ensure ethical deployment, compliance with healthcare standards, and patient data protection. Pilot projects and phased implementations can help providers assess AI’s impact on workflows and patient care.
What is the future outlook for AI in medical imaging?
The future of AI in medical imaging looks promising with advancements in machine learning capabilities, enhanced image analysis tools, and integration with emerging technologies like cloud computing and IoT. Continued research, regulatory clarity, and investments in AI infrastructure are key to realizing its full potential in improving healthcare outcomes globally.
Conclusion
AI is transforming medical imaging by enhancing diagnostic accuracy, efficiency, and personalized patient care. While there are challenges to address, the benefits of AI in healthcare are substantial, paving the way for a future where advanced technologies support clinicians in providing better outcomes for patients worldwide.
This FAQ section covers essential questions about AI in medical imaging, providing detailed answers and links to relevant resources for further reading. Adjustments can be made based on specific topics or additional queries you’d like to include.
Disclaimer and Caution: AI in Medical Imaging
Disclaimer
The integration of Artificial Intelligence (AI) in medical imaging represents a significant advancement in healthcare technology, offering potential benefits in diagnostic accuracy, efficiency, and personalized patient care. However, it is crucial to understand and acknowledge certain disclaimers and cautions associated with the use of AI in this critical domain.
Limitations of AI in Medical Imaging
1. Data Dependence: AI algorithms depend heavily on the quality and diversity of the data used for training. Variations in data sources, such as demographics and imaging protocols, can affect algorithm performance and diagnostic accuracy.
2. Algorithm Bias: AI models may exhibit biases inherent in the training data, leading to disparities in diagnostic outcomes across different patient populations. Recognizing and mitigating algorithmic biases are ongoing challenges in AI development.
3. Regulatory Compliance: Healthcare regulations and standards often lag behind rapid technological advancements in AI. Ensuring compliance with regulatory requirements and ethical guidelines is essential to safeguard patient rights and ensure safe and effective use of AI technologies in medical practice.
4. Validation and Interpretation: While AI can assist in image interpretation, final diagnostic decisions should always be made by qualified healthcare professionals. AI outputs should be interpreted in the context of clinical expertise and patient-specific factors to avoid misdiagnosis or improper treatment.
Cautionary Considerations
1. Privacy and Data Security: The use of AI in medical imaging involves handling large volumes of sensitive patient data. Healthcare providers and technology developers must prioritize data privacy, security measures, and compliance with legal requirements (such as HIPAA in the United States) to protect patient confidentiality.
2. Continuous Monitoring and Maintenance: AI algorithms require ongoing monitoring and maintenance to ensure their reliability and performance. Regular updates, validation studies, and adherence to best practices in algorithm management are essential to minimize risks and optimize outcomes.
3. Ethical Use and Transparency: Transparent communication regarding the capabilities, limitations, and potential risks of AI systems in medical imaging is crucial for building trust among patients, healthcare providers, and regulatory authorities. Ethical considerations should guide the development, deployment, and evaluation of AI technologies to promote equitable healthcare delivery.
4. Collaborative Approach: Successful integration of AI in medical imaging requires collaboration among multidisciplinary teams, including radiologists, data scientists, ethicists, and policymakers. Stakeholder engagement and knowledge sharing are essential for addressing complex challenges and maximizing the benefits of AI in healthcare.
Conclusion
In conclusion, while AI holds promise in revolutionizing medical imaging with enhanced diagnostic capabilities and efficiency gains, it is essential to approach its adoption with caution and awareness of potential limitations and challenges. By addressing these considerations through rigorous validation, ethical practices, and collaborative efforts, AI technologies can contribute to advancing healthcare outcomes responsibly and ethically.
This disclaimer and caution section provides a comprehensive overview of the considerations and risks associated with the use of AI in medical imaging, emphasizing the importance of ethical deployment, regulatory compliance, and continuous monitoring for safe and effective healthcare practices. Adjustments can be made based on specific requirements or additional topics you’d like to include.
1 thought on “The Future of Medical Imaging”