The Role of AI in Predictive Healthcare: Tools and Applications
Introduction to Predictive Healthcare
The integration of Artificial Intelligence (AI) into healthcare has revolutionized the way we approach medical treatment and disease prevention. Predictive healthcare leverages AI to anticipate health events and provide personalized care, enhancing outcomes and efficiency.
AI Tools in Predictive Healthcare
AI tools are at the forefront of predictive healthcare, employing complex algorithms and machine learning models to analyze vast datasets. Key tools include:
Tool | Description | Applications |
---|---|---|
Machine Learning | Utilizes algorithms to learn from data and make predictions. | Predicting patient outcomes, identifying high-risk patients. |
Natural Language Processing (NLP) | Analyzes and understands human language data. | Extracting insights from clinical notes, automating patient interactions. |
Deep Learning | Advanced machine learning technique using neural networks. | Image analysis, genomics, drug discovery. |
Predictive Analytics | Statistical techniques to forecast future outcomes. | Preventative care, resource management. |
Applications of AI in Predictive Healthcare
AI applications in predictive healthcare are vast and varied, impacting numerous areas:
- Disease Prediction: AI models predict disease outbreaks and individual susceptibility, aiding in early intervention and prevention. For example, AI algorithms can forecast flu outbreaks by analyzing search engine data and social media trends.
- Personalized Medicine: AI tailors treatments based on individual genetic profiles, improving the efficacy of interventions and reducing adverse effects.
- Resource Allocation: Predictive analytics helps in efficient allocation of medical resources, such as hospital beds and ventilators, by predicting demand spikes.
- Patient Monitoring: AI-driven wearables and remote monitoring devices continuously track patient health metrics, enabling proactive healthcare management.
Success Stories and Exemplary Cases
Numerous success stories highlight the transformative impact of AI in predictive healthcare:
One prominent example is IBM Watson Health, which uses AI to assist in diagnosing diseases and suggesting treatment options, significantly enhancing clinical decision-making.
Another success story is Google Health, leveraging AI for disease detection and management, including projects on diabetic retinopathy and breast cancer detection.
Challenges and Considerations
While the benefits of AI in predictive healthcare are substantial, several challenges must be addressed:
- Data Privacy: Ensuring the confidentiality and security of patient data is paramount. Striking a balance between data utility and privacy is critical.
- Bias and Fairness: AI models can perpetuate existing biases present in training data, leading to unfair treatment outcomes. Continuous monitoring and bias mitigation strategies are essential.
- Integration: Seamlessly integrating AI tools with existing healthcare systems and workflows requires significant effort and collaboration among stakeholders.
- Regulation: Establishing comprehensive regulatory frameworks that keep pace with rapid AI advancements is necessary to ensure safety and efficacy.
The Future of AI in Predictive Healthcare
The future of AI in predictive healthcare is promising, with ongoing advancements set to further revolutionize the field:
Emerging technologies such as quantum computing could enhance AI’s predictive capabilities, enabling even more accurate and timely interventions.
Increased collaboration between technology companies and healthcare providers will drive innovation, resulting in more integrated and effective AI solutions.
With continuous improvements in data collection and analysis, AI will become an indispensable tool in predictive healthcare, ultimately leading to better patient outcomes and more efficient healthcare systems.
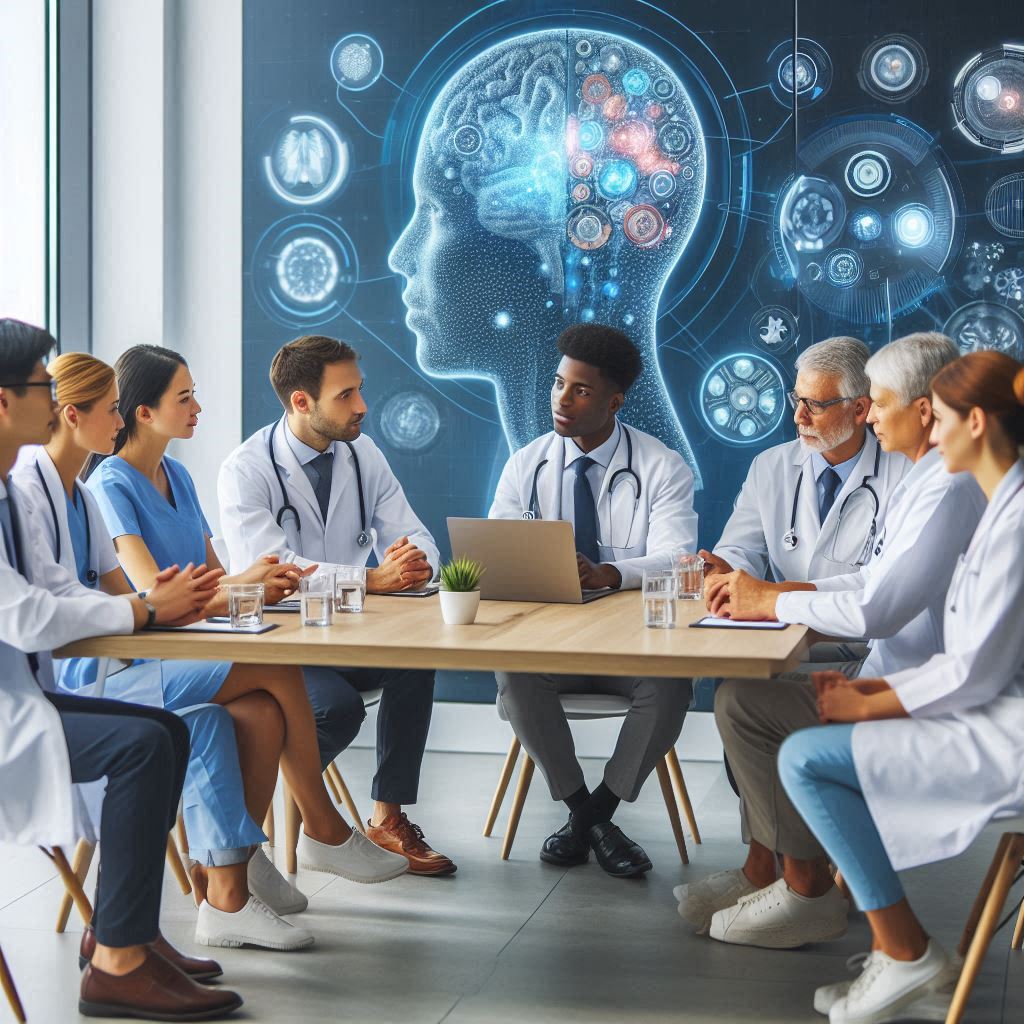
Conclusion
AI’s role in predictive healthcare is transformative, offering unprecedented opportunities for enhancing patient care and system efficiency. As the technology evolves, addressing challenges such as data privacy, bias, and regulatory compliance will be crucial to fully realize its potential. The ongoing collaboration between the tech industry and healthcare providers promises a future where AI-driven predictive healthcare becomes the norm, leading to a healthier world.
References
- IBM Watson Health
- Google Health
- Artificial Intelligence in Healthcare
- Predictive Analytics in Healthcare
Pros and Cons in Detail
Pros of AI in Predictive Healthcare
- Enhanced Disease Prediction
- AI algorithms can sift through large datasets, recognizing patterns that may escape human analysts. For instance, predictive models can forecast flu outbreaks by analyzing data from social media platforms and search engines. This capability allows for timely public health interventions, reducing the potential spread of infectious diseases.
- Personalized Medicine
- AI empowers healthcare professionals to tailor treatments to individual patients by analyzing genetic, environmental, and lifestyle factors. For example, precision oncology utilizes AI to customize cancer treatments based on specific genetic mutations, leading to more effective therapies and minimized side effects.
- Improved Patient Monitoring
- With the rise of wearable technologies, AI facilitates continuous monitoring of patient health metrics. Devices like smartwatches can track heart rate, physical activity, and glucose levels, allowing for proactive management of chronic conditions such as diabetes and hypertension.
- Efficient Resource Allocation
- AI-driven predictive analytics can help hospitals and clinics anticipate demand for services and resources. For instance, during a pandemic, AI models can predict ICU bed needs based on patient admission trends, enabling better planning and allocation of resources.
- Streamlined Clinical Workflows
- AI automates various administrative tasks, such as scheduling appointments and managing billing processes, thus freeing up healthcare professionals to focus more on patient care. This automation can lead to enhanced operational efficiency within healthcare systems.
- Advanced Diagnostics
- AI technologies, particularly in medical imaging, significantly enhance diagnostic accuracy. AI algorithms can analyze radiological images to detect anomalies like tumors or fractures with remarkable precision, contributing to early and accurate disease detection.
Cons of AI in Predictive Healthcare
- Data Privacy Concerns
- The deployment of AI in healthcare necessitates the collection of vast amounts of personal health data, raising significant privacy concerns. Data breaches or misuse can lead to unauthorized access to sensitive information, undermining patient trust and compliance with health regulations.
- Bias and Fairness Issues
- AI systems can perpetuate biases present in training datasets, leading to unequal treatment outcomes. For example, an AI model trained predominantly on data from one demographic may perform poorly when applied to others, exacerbating health disparities.
- Integration Challenges
- Incorporating AI systems into existing healthcare infrastructures can pose significant challenges. Compatibility issues with legacy systems and the complexity of integrating new technologies can result in high implementation costs and resistance from healthcare providers.
- Regulatory and Ethical Dilemmas
- The rapid pace of AI advancement often outstrips the development of regulatory frameworks, creating legal ambiguities around AI usage in clinical settings. Establishing guidelines that ensure the safe and ethical application of AI in healthcare is essential but often lagging.
- Dependence on Technology
- There is a risk that healthcare professionals may become overly reliant on AI systems, potentially diminishing their clinical judgment. This dependence can lead to reduced critical thinking skills among clinicians and a decreased capacity for independent decision-making.
- High Initial Costs
- Implementing AI technologies requires substantial financial investment in software development, training, and infrastructure. Smaller healthcare providers may face significant financial barriers to adopting AI solutions, leading to disparities in access to advanced healthcare technologies.
Conclusion
The integration of AI in predictive healthcare presents a promising yet complex landscape. While the potential benefits are significant, addressing the challenges is crucial to ensure that AI contributes positively to the future of healthcare.
FAQs: The Role of AI in Predictive Healthcare
1. What is predictive healthcare?
Predictive healthcare refers to the use of data analysis and AI technologies to forecast health outcomes and disease occurrences. By analyzing historical and real-time data, predictive models can identify trends and predict individual and population health risks.
Learn more: What is Predictive Healthcare?
2. How does AI contribute to disease prediction?
AI contributes to disease prediction by utilizing machine learning algorithms to analyze large datasets, identifying patterns that indicate the likelihood of disease outbreaks or patient risks. For example, AI can analyze social media trends or electronic health records to predict influenza outbreaks.
Learn more: AI in Disease Prediction
3. What are some examples of AI tools used in predictive healthcare?
Several AI tools are pivotal in predictive healthcare, including:
Tool | Description | Applications |
---|---|---|
Machine Learning | Algorithms that learn from data | Disease prediction, patient risk assessment |
Natural Language Processing (NLP) | Analyzes human language data | Clinical note analysis, patient interactions |
Deep Learning | Advanced neural networks for data processing | Imaging analysis, genomics |
Predictive Analytics | Statistical techniques to forecast outcomes | Preventative care, resource management |
Learn more: AI Tools in Healthcare
4. What are the benefits of AI in personalized medicine?
AI enhances personalized medicine by analyzing genetic information, lifestyle factors, and environmental influences to tailor treatments to individual patients. This leads to:
- Higher treatment efficacy
- Reduced side effects
- Customized care strategies
Learn more: Personalized Medicine and AI
5. How does AI improve patient monitoring?
AI improves patient monitoring through real-time data analysis from wearable devices and remote monitoring systems. These technologies continuously track vital signs, alerting healthcare providers to potential health issues before they escalate.
Example: Continuous glucose monitors for diabetes management.
Learn more: AI in Patient Monitoring
6. What challenges does AI face in predictive healthcare?
Despite its advantages, AI in predictive healthcare encounters several challenges:
- Data Privacy Concerns: Safeguarding patient data is critical due to the potential for breaches.
- Bias and Fairness Issues: AI systems may reflect biases present in training data, leading to unequal outcomes.
- Integration Challenges: Compatibility issues with existing healthcare systems can hinder implementation.
- Regulatory and Ethical Dilemmas: Rapid AI advancement often outpaces regulatory frameworks.
Learn more: Challenges in AI Healthcare
7. How does AI ensure efficient resource allocation in healthcare?
AI uses predictive analytics to forecast healthcare resource needs, allowing institutions to prepare for fluctuating demands. For example, during a health crisis, AI models can predict ICU bed requirements and optimize resource distribution accordingly.
Learn more: Resource Allocation and AI
8. What are the risks associated with over-reliance on AI in healthcare?
Over-reliance on AI may lead to diminished clinical judgment and critical thinking skills among healthcare professionals. There’s a risk that clinicians may defer excessively to AI recommendations rather than applying their expertise.
Learn more: Risks of AI in Healthcare
9. What role does regulatory oversight play in AI healthcare applications?
Regulatory oversight is crucial in ensuring that AI applications in healthcare are safe, effective, and ethical. Clear guidelines are necessary to address concerns regarding data privacy, bias, and the overall efficacy of AI systems in clinical settings.
Learn more: AI Regulation in Healthcare
10. Can AI in healthcare perpetuate biases, and how can this be addressed?
Yes, AI can perpetuate biases if the training datasets reflect existing inequalities. To address this, continuous monitoring, diverse data sourcing, and bias mitigation strategies are essential in the development and implementation of AI systems.
Learn more: Addressing Bias in AI
11. What are the financial implications of adopting AI in healthcare?
Adopting AI in healthcare can require significant initial investment in technology, training, and infrastructure. While the long-term benefits often outweigh these costs, smaller healthcare providers may face financial barriers to entry.
Learn more: Financial Implications of AI
12. How can AI enhance clinical workflows?
AI enhances clinical workflows by automating routine administrative tasks such as scheduling, billing, and patient record management. This automation reduces the administrative burden on healthcare professionals, allowing them to focus more on patient care.
Learn more: AI in Clinical Workflows
13. What is the future of AI in predictive healthcare?
The future of AI in predictive healthcare is promising, with ongoing advancements set to improve diagnostic accuracy, personalize patient care, and streamline healthcare processes. Emerging technologies, such as quantum computing, are expected to further enhance AI’s capabilities.
Learn more: Future Trends in AI Healthcare
14. Are there notable success stories of AI in predictive healthcare?
Yes, several notable success stories highlight the transformative impact of AI:
- IBM Watson Health: Assists in diagnosing diseases and recommending treatment options, significantly enhancing clinical decision-making. Learn more
- Google Health: Utilizes AI for disease detection and management, including projects focused on diabetic retinopathy. Learn more
Learn more about these initiatives: AI Success Stories in Healthcare
15. What ethical considerations surround the use of AI in healthcare?
Ethical considerations surrounding AI in healthcare include:
- Ensuring patient autonomy in decision-making.
- Maintaining transparency in AI algorithms and their decision processes.
- Addressing biases to avoid unequal treatment outcomes.
Learn more: Ethics in AI Healthcare
Conclusion
AI’s role in predictive healthcare continues to evolve, presenting both significant opportunities and challenges. Understanding these aspects is crucial for stakeholders in the healthcare sector to harness AI’s full potential while addressing associated risks.
Disclaimer and Caution
Overview
The integration of Artificial Intelligence (AI) into predictive healthcare represents a significant advancement in medical technology and patient care. However, while the potential benefits are substantial, it is essential to understand the limitations, risks, and ethical considerations associated with AI applications in this critical field. This disclaimer serves to outline these important factors for healthcare professionals, researchers, patients, and policymakers.
1. General Disclaimer
The information presented regarding the role of AI in predictive healthcare is intended for educational purposes only. It does not constitute medical advice, diagnosis, or treatment. Readers are encouraged to consult with qualified healthcare professionals for personalized medical advice tailored to their individual health needs and circumstances.
2. Limitations of AI Technologies
a. Data Dependency
AI systems rely heavily on data quality and availability. The effectiveness of predictive models is directly tied to the quality of the input data, which can vary widely across different populations and settings.
- Implication: Incomplete or biased data can lead to inaccurate predictions, potentially compromising patient care.
b. Algorithmic Transparency
Many AI models operate as “black boxes,” meaning their internal workings are not always transparent or understandable to users, including healthcare professionals.
- Implication: Lack of transparency can hinder trust and complicate clinical decision-making, as clinicians may not fully understand how a recommendation is derived.
c. Contextual Limitations
AI tools are often trained on specific datasets that may not represent the full spectrum of patient populations or clinical scenarios.
- Implication: The performance of AI systems may decline when applied to populations or contexts that differ from the training data, leading to potential biases in clinical outcomes.
3. Potential Risks and Ethical Considerations
a. Data Privacy and Security
The use of AI in healthcare requires the collection and analysis of vast amounts of sensitive patient data, raising significant privacy and security concerns.
- Implication: There is a risk of data breaches or misuse, which can erode patient trust and violate privacy regulations.
b. Bias and Fairness
AI algorithms can inadvertently perpetuate existing biases present in the training datasets, leading to unequal treatment outcomes across different demographic groups.
- Implication: Failure to address bias can result in disparities in healthcare delivery, exacerbating existing inequalities within the healthcare system.
c. Ethical Dilemmas
The rapid advancement of AI in healthcare poses several ethical challenges, including questions around accountability, informed consent, and the role of human oversight.
- Implication: Determining who is responsible for decisions made by AI systems—whether the developers, healthcare providers, or institutions—can be complex and contentious.
4. Regulatory and Compliance Issues
a. Evolving Regulatory Landscape
The regulatory framework surrounding AI in healthcare is continuously evolving and may not yet fully address the unique challenges posed by AI technologies.
- Implication: Healthcare providers and organizations must stay informed about the latest regulations and ensure compliance to avoid legal and ethical pitfalls.
b. Need for Validation and Certification
AI tools must undergo rigorous validation and certification processes to ensure their safety and efficacy in clinical settings.
- Implication: Utilizing unvalidated or uncertified AI systems can lead to serious risks, including misdiagnosis or inappropriate treatment recommendations.
5. Clinical Decision-Making and Human Oversight
a. Augmentation, Not Replacement
AI is intended to augment, not replace, the clinical decision-making processes of healthcare professionals. Human expertise and judgment remain vital components of effective patient care.
- Implication: Clinicians should always critically evaluate AI-generated recommendations and consider the broader clinical context before making decisions.
b. Risk of Over-reliance
There is a potential risk that healthcare providers may become overly reliant on AI systems, diminishing their clinical intuition and critical thinking skills.
- Implication: It is crucial for healthcare professionals to maintain their analytical skills and not defer completely to AI recommendations.
6. Financial Implications and Accessibility
a. High Implementation Costs
Implementing AI technologies in healthcare can require substantial financial investments in infrastructure, training, and ongoing maintenance.
- Implication: Smaller healthcare providers may face significant financial barriers to entry, leading to disparities in access to advanced AI solutions.
b. Long-term Cost-Benefit Analysis
While AI can lead to cost savings in the long run, the initial investment and uncertainty around ROI can pose challenges for healthcare organizations.
- Implication: Stakeholders must conduct thorough cost-benefit analyses to assess the viability of AI implementations.
7. Future Considerations and Uncertainties
a. Rapid Technological Advancements
The field of AI is rapidly evolving, with new technologies and applications emerging frequently. This dynamic landscape can create uncertainty around the best practices and tools to adopt.
- Implication: Healthcare organizations must be prepared to adapt to ongoing changes and continuously evaluate their AI strategies.
b. Potential Job Displacement
The increased automation of tasks traditionally performed by healthcare professionals could lead to concerns about job displacement and workforce dynamics.
- Implication: Stakeholders must consider the broader implications of AI adoption on the healthcare workforce and address potential challenges proactively.
8. Liability and Accountability
a. Legal Implications
The use of AI in healthcare raises complex legal questions regarding liability and accountability in the event of adverse outcomes.
- Implication: Determining liability in cases involving AI-generated recommendations or decisions can be legally challenging and requires careful consideration.
b. Need for Clear Guidelines
Establishing clear guidelines and frameworks for the ethical use of AI in healthcare is essential to navigate potential legal issues and ensure accountability.
- Implication: Stakeholders must engage in ongoing dialogue to develop consensus on best practices and accountability standards.
9. Patient Involvement and Informed Consent
a. Engaging Patients in AI Usage
Patients should be involved in discussions regarding the use of AI in their healthcare and informed about the potential benefits and risks associated with AI technologies.
- Implication: Transparent communication can foster trust and ensure that patients are active participants in their care.
b. Informed Consent Challenges
Obtaining informed consent for the use of AI in healthcare may be complex, particularly when patients may not fully understand AI technologies and their implications.
- Implication: Healthcare providers must strive to communicate effectively and ensure patients comprehend the nature and purpose of AI applications.
10. Final Thoughts
The integration of AI in predictive healthcare holds tremendous potential to enhance patient care, improve outcomes, and streamline processes. However, stakeholders must approach these advancements with caution, recognizing the limitations, risks, and ethical considerations involved. A balanced perspective that prioritizes patient safety, ethical standards, and regulatory compliance will be essential for harnessing the full benefits of AI in healthcare.
Disclaimer Summary
- Not Medical Advice: This information is for educational purposes and does not substitute for professional medical advice.
- Continuous Evaluation: AI technologies should be continually assessed for efficacy, bias, and relevance to clinical practice.
- Informed Decision-Making: All stakeholders must engage in informed decision-making processes when implementing AI solutions in healthcare settings.
By understanding these nuances, stakeholders can navigate the complexities of AI in predictive healthcare and contribute to a safer, more equitable healthcare landscape.
6 thoughts on “The Role of AI in Predictive Healthcare”